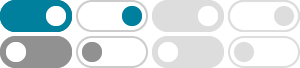
Stochastic differential equation - Wikipedia
A stochastic differential equation (SDE) is a differential equation in which one or more of the terms is a stochastic process, [1] resulting in a solution which is also a stochastic process. SDEs have many applications throughout pure mathematics and are used to model various behaviours of stochastic models such as stock prices , [ 2 ] random ...
In this lecture, we study stochastic di erential equations. See Chapter 9 of [3] for a thorough treatment of the materials in this section. 1. Stochastic differential equations. for given functions a and b, and a Brownian motion B(t). A function (or a path) X is a solution to the di erential equation above if it satis es.
Trajectory of the differential equation Notation. x(t) is the state of the system at time t≥ 0, x˙(t) := d dt x(t). In many applications, however, the experimentally measured trajectories of systems modeled by (ODE) do not in fact behave as predicted: X(t) x0 Sample path of the stochastic differential equation
Stochastic Differential Equations (SDE) In general an SDE is given as dX(t,ω) = f(t,X(t,ω))dt + g(t,X(t,ω))dW(t,ω), (7) where ω denotes that X = X(t,ω) is a random variable and possesses the initial condition X(0,ω) = X0 with probability one. As an example we have already encountered dY (t,ω) = µ(t)dt + σ(t)dW(t,ω).
tion of an associated Ito difiusion (i.e. solution of a stochastic difierential equation) leads to a simple, intuitive and useful stochastic solution, which is the cornerstone of stochastic potential theory.
Many important continuous-time Markov processes — for instance, the Ornstein-Uhlenbeck pro-cess and the Bessel processes — can be defined as solutions to stochastic differential equations with drift and diffusion coefficients that depend only on the current value of the process.
Many important continuous-time Markov processes — for instance, the Ornstein-Uhlenbeck pro-cess and the Bessel processes — can be defined as solutions to stochastic differential equations with drift and diffusion coefficients that depend only on the current value of the process.
Equations with multiplicative noise have to be treated more carefully then equations with additive noise. We learned how to define the integrals in the expressions above last class. In this one we’ll look at properties of the solutions themselves. We will ask: when do solutions exist? Are they unique? And how can we
LECTURE 12: STOCHASTIC DIFFERENTIAL EQUATIONS, DIFFUSION PROCESSES, AND THE FEYNMAN-KAC FORMULA 1. Existence and Uniqueness of Solutions to SDEs It is frequently the case that economic or nancial considerations will suggest that a stock price, exchange rate, interest rate, or other economic variable evolves in time according to a stochastic
Abstract. This paper introduces stochastic calculus and stochastic differen-tial equations. We start with basic stochastic processes such as martingale and Brownian motion. We then formally define the Itˆo integral and establish Itˆo’s formula, the fundamental theorem of stochastic calculus. Finally, we prove